In this episode, VibeCast host Ray Dogum speaks with Alex Zhavoronkov, PhD, founder and CEO of Insilico Medicine, about his journey starting the company in 2014. Insilico Medicine’s mission is to extend healthy, productive longevity by transforming drug discovery and development with generative artificial intelligence.
Aging research and drug development in the era of AI
In the world of drug discovery, artificial intelligence (AI) has become a game-changer, revolutionizing how pharmaceutical companies develop new medications. AI algorithms can analyze massive datasets to identify potential drug candidates faster and more accurately than traditional methods. This advancement has significantly accelerated the drug discovery process, allowing for the exploration of a wider range of compounds and potential treatments.
Alex Zhavoronkov, PhD sheds light on the transformative power of AI in drug discovery during his conversation with VibeCast host, Ray Dogum. He discusses how AI, particularly deep neural networks, is being utilized to sift through vast amounts of biological data to identify promising compounds for various diseases. Alex’s insights emphasize the critical role of AI in not only streamlining drug development but also in potentially uncovering novel treatments that may have been overlooked using conventional approaches. His expertise underscores the immense potential of AI to reshape the pharmaceutical industry and improve global healthcare outcomes.
In the discussion, Alex shares personal anecdotes and professional milestones that shaped his career trajectory in longevity research. By embracing interdisciplinary learnings and immersing himself in the complexities of biopharma, he demonstrates how individuals can contribute significantly to transformative advancements in drug development. Alex’s journey underscores the importance of persistence, adaptability, and a relentless pursuit of knowledge in driving innovation in the biotech industry.
Watch VibeCast on YouTube
Key moments
- 00:00:08 – Introduction
- 00:01:14 – Alex Zhavoronkov’s Journey from Computer Science to Longevity Research
- He explains his transition from computer science to biotech longevity, emphasizing the unfairness of aging and his passion for continuous improvement in health.
- 00:05:24 – Application of Deep Neural Networks in Biomedical Research
- Alex delves into the application of deep neural networks in aging research and drug discovery. He highlights the synchronization of biological data types with time and the use of generative adversarial networks for predicting and imagining new molecules with desired properties.
- 00:08:33 – Overcoming Challenges in AI Adoption in Biotech
- Alex discusses the challenges faced in the early days of AI adoption in biotech, including skepticism from the industry, the slow pace of collaborations, and the importance of scalability and proof of concept. He also emphasizes the need for passion and hard work to deliver results.
- 00:17:07 – Ensuring Ethical and Unbiased AI in Healthcare
- Alex emphasizes the rigorous regulatory standards in biopharma to ensure ethical and unbiased
- 00:18:32 – Importance of Safety in Drug Development
- Alex discusses the importance of proving safety and efficacy before starting clinical trials and the challenges in demonstrating drug safety, especially for chronic diseases.
- 00:21:53 – FDA Regulations and Drug Approval
- Alex shares his perspective on the robustness of FDA regulations and the need for more evidence to support the capability of AI-designed drugs for faster approvals.
- 00:26:42 – Global Collaboration and Opportunities in Africa
- Alex emphasizes the potential for global collaboration to accelerate drug approvals and set records, especially for rare diseases where patients are in urgent need of treatment.
- 00:30:03 – Democratization of AI Technology
- Alex discusses the potential of AI to benefit developing nations and regions with limited resources by providing access to educational capabilities and democratizing technology for drug discovery.
- 00:35:18 – Future of Biotech and AI
- Alex shares his excitement about generative approaches, robotics, quantum computing, and regenerative medicine, highlighting the potential for comprehensive life simulations and the ethical implications of growing new organs.
- 00:37:22 – The Future of Longevity Research
- Alex discusses the direction of longevity research and the potential for innovative treatments like new organs to cure diseases like kidney cancer.
- 00:37:54 – Psychological Aging
- The concept of psychological aging is highlighted as the most influential factor in determining one’s health and behavior. Convincing oneself of a longer lifespan can lead to a healthier and more youthful lifestyle.
- 00:38:22 – Conclusion and Call to Action
- Ray thanks Alex for the insightful conversation and encourages the audience to like, share, and subscribe to the podcast. The audience is encouraged to adopt a mindset of longevity and share the knowledge with others.
Also available on Spotify
Guest Biography
Alex Zhavoronkov, PhD, is the founder and CEO of Insilico Medicine (insilico.com), a leading clinical-stage biotechnology company developing next-generation generative artificial intelligence and robotics platforms for drug discovery. Since 2014, he has invented critical technologies in the field of generative artificial intelligence and reinforcement learning (RL) for the generation of novel molecular structures with the desired properties and the generation of synthetic biological and patient data. He also pioneered the applications of transformers and other deep learning technologies for the prediction of human biological age using multiple data types, transfer learning from aging into disease, target identification, and signaling pathway modeling. Under his leadership, Insilico raised over $400 million in multiple rounds from expert biotechnology, healthcare, and financial investors, opened R&D centers in 6 countries and regions, and partnered with multiple pharmaceutical, biotechnology, and academic institutions. Since 2021, the company nominated 18 preclinical candidates, started 6 human clinical trials, and entered Phase II with an AI-discovered novel target and AI-designed novel molecule. By 2023, 11 out of the top 20 pharmaceutical companies used a part of the Pharma.AI software suite, and the IND-stage cancer program was sold in a deal with $80 million upfront as a testament to the quality and novelty of the AI-generated molecule.
Prior to founding Insilico, he worked in senior roles at ATI Technologies (GPU company acquired by AMD). Since 2012, he has published over 200 peer-reviewed research papers with over 30 papers in the field of generative adversarial networks, generative reinforcement learning, and multi-modal transformers, and 3 books, including “The Ageless Generation: How Biomedical Advances Will Transform the Global Economy” (Macmillan, 2013). He serves on the advisory or editorial boards of Trends in Molecular Medicine, Aging Research Reviews, Aging, and Frontiers in Genetics, and founded and co-chairs the Annual Aging Research and Drug Discovery (10th Annual in 2023), the world’s largest event on aging research in the biotechnology industry. He is the adjunct professor of artificial intelligence at the Buck Institute for Research on Aging.
Guest Links
Full Transcript
Note: This podcast was partially edited and transcribed using AI technology. While we strive for accuracy, some errors or inconsistencies may occur due to the automated process.
Ray: Hello and welcome to VibeCast by Vibe Bio, where we aim to find every cure for every community with the help of AI. I’m your host, Ray Dogum. In this episode, our guest is Dr. Alex Zhavoronkov founder and CEO of Insilico Medicine, a leader in using AI and robotics for drug discovery. Alex has published over 200 peer reviewed research papers with over 30 papers in the field of generative adversarial networks, generative reinforcement learning, and multimodal transformers.
He’s written three books including The Ageless Generation: How Biomedical Advances Will Transform the Global Economy. He also founded and co chairs the annual aging research and drug discovery conference, the world’s largest event on aging research in the biotech industry. Alex, it’s such an honor to be speaking with you today.
How’s your day going?
Alex: Great to be with you. The day never ended since yesterday. So to put a little bit of an all nighter but I hope that what you’re going to see from us on the next A few days is going to be pretty epic.
Ray: That’s really exciting. So I want to set the stage for the audience to understand really who you are and what your background is.
I understand you have a computer science background, but then you started becoming interested in the longevity space. So can you talk to us about your journey? From computer science into biotech longevity.
Alex: Oh, sure. I’ve been interested in aging research all my life because I don’t think it’s fair to stop continuously improving at some point in time and just start declining and decaying regardless of how hard you work.
Nature is very unfair. And at the very least, we need to figure out how to keep it, at the same level or declined without significant diseases. But I was born during kind of the great turbulent time in Latvia. And at that time, in that region the entire kind of science collapsed.
So I had to go to Canada and I became Canadian and started working in computer science originally. So I did my first two degrees in computer science in commerce at Queen’s University in Canada. I worked for a number of semiconductor companies. So one of those semiconductor companies was ATI Technologies.
At that time, it was a leader in GPU. Those are the semiconductors that are powering deep neural networks today. And GPGPU was all general purpose graphics processing units were already pretty popular at the time. I was looking into that. I was also playing the stock market from time to time and made a reasonable amount of money in my early 20s.
So enough to sustain myself for a little bit. I decided to go and do my graduate work at Johns Hopkins University and my technology mathematics target discovery. And then worked for a number of biotechs had my own lab and even worked for a conference organizing company just to learn many kind of different phases of biotech oncology, drug discovery.
molecular targets stem cell biology organized many events and then had again, my own lab and regenerative medicine, bioinformatics. And then at the dawn of the deep learning revolution when deep neural networks just starting, started to showcase some of their capabilities. So 2012, 2013.
I started going to NVIDIA GTC conferences by the invitation of my colleagues who migrated from ATI to NVIDIA. And I started presenting there, right? So we presented in SILIC deep learning for drug discovery and aging research.
Ray: And this is back in 2013, 2014.
Alex: Yeah, 2014. So Phil Eisler, my colleague from ATI, now works for NVIDIA, invited me and actually said, Alex, you have to come, right?
This is the time. I’m deeply thankful to him for doing that. And I come back to Baltimore and I just decided to start Insilico. I thought, okay this is the time because deep neural networks are performing so well in voice recognition, image recognition and now why don’t we start training deep neural networks on different biological data types?
So something that humans cannot see, so what they also those biological data types change in time in a very similar ways as a video, right? So our our life is, of course, a collection of pictures, right? But it’s also, you can turn it into a continuous video flow and you can train deep neural networks to understand the different features that Layer that depend on each other form patterns and connect the previously disconnected data types, right?
So for example before if you’re working with expression of genes, gene expression, so you know how biology is organized, so I’ve got a DNA DNA is read into RNA, so that’s gene expression, and then RNA becomes protein expression. So if you are working with, let’s say, gene expression data, you would usually build a single purpose statistical model or some primitive machine learning model to predict something.
But we realized that now you can actually train deep neural networks on gene expression, on protein expression, and methylation at the same time to predict one feature that everybody has, and that feature is age. We are all different. We some of us have cancers, some of them, some of us don’t.
We are very unique. But what unites us is actually time. So we can just like with a synchronous optical network, you can find the clock, right? And we are the entire network on the clock. So we are synchronized in time and you can now connect. previously disconnected data types into models that understand biology in time.
And we started focusing on that so published a few of those aging clocks. My first aging clock using deep learning was published 2016. And at the same time, I got very interested in the work of Ian Goodfellow and Yoshua Bengio, who proposed the concept of generative adversarial networks, where you can not only predict, but also imagine new things.
With the desired properties and our first paper in the generation of novel molecules with the desired properties were also those papers were published 2016 and then we switched to other models on increased the number of different models and created a Lego system that allows you to understand biology, but also chemistry, create new drugs and get those drugs validated.
And then we actually started discovering and developing those drugs. So that’s the pathway over the past 10 years. So starting from biology AI, then we went to chemistry AI. Then we launched software in the market and started developing our own drugs. Yeah.
Ray: And you have at least 31 programs drug programs that were developed using this technology.
That’s quite impressive. And of course it took a lot of, many years you started this company inSilico Medicine in 2014. This is before ChatGPT, before this idea of everyone being able to, generate art and images and all these things. Has there been this sort of aha moment in the community where now everyone does believe in AI?
Because I’m assuming back then getting clients and getting, People to work with you was challenging, I would say it was
Alex: more than challenging because when you’re entering this industry, and this is the industry where everybody knows each other and people really value backgrounds. So if you come in with a background that.
does not, that is not representative of your typical biotechnology CEO. It was very difficult to partner, right? Or if you don’t come with a top tier brand, you would say come from a position at Google, right? Or or some other big company that pioneered artificial intelligence when Google actually is the most Impactful if you look back, right?
Nowadays, of course, you’ve got a lot of different companies, right? And Google became sort of Xerox where a lot of companies,
Ray: potentially in the future.
Alex: I think there are there are a little bit, right? So Microsoft did the impossible, right? And, open AI and many other companies and know what X dot AI is doing right now.
It’s pretty incredible things. So a lot of entrance started performing much better on the applied side than the people who actually put the theory together and made sure that it’s available to the world. So deep bow to Google, but of course on the applied side This guy started companies like Calico, for example, it also went with AI after aging.
Where are the drugs, right? There are after all those billions of investments. So it really requires a lot of passion and really hard work to, deliver. And in the early days when we just started, even deep learning was very people were skeptical that it was going to perform really well in biotech.
And every time you submit a research publication, you always have to compare with traditional approaches, right? You compare with linear regression, you compare with support vector machines, so more classical forms of machine learning here in k nearest neighbors, and very often those models outperform in domain specific tasks, right?
And especially when the data is limited. That’s it. So you have to explain that it’s all about proof of concept scalability. And some journals understood, some didn’t, especially, again, if you don’t come with a big name. And pharma companies did the same, right? More classical computational research folks, who were the incumbents, right?
And they started learning, deep learning, but they were also skeptical at the time. They started doing what was called pilots. People still like to do pilots, right? Whenever you have the technology that is yet to be proven they give you a little bit of money, sometimes even no money. And you partner on testing your technology with their problem.
And very often you don’t even know what the problem is until you sign. And you have to sign a lot of CDAs, et cetera. Sometimes that process took months if not years, to do a partnership, do a pilot. So the pace of those collaborations was much slower than what you typically would expect.
And we’re left through the period that I like to call death by pilot, right? So you complete multiple pilots, very often you outperform. But by the time you complete the pilot, the technology has moved on. And people want a new shiny thing and they want another pilot. So until you realize that you have to just do it yourself, right?
And give them the tool, it’s going to be all pilots, right? And very often in the pharmaceutical industry, management changes right? So people travel from one company to another. You lose your contact and they appear somewhere else where they still need to establish themselves. You lose the program.
So this is this was unsustainable. And also, at the same time, when you presented that conferences, especially medicinal chemistry conferences, in my opinion, medicinal chemists are probably the smartest humans on the planet, right? Because they have to understand so many things at once, right? So they have the more kind of umbrella view on drug discovery than any other function within the organization.
They have to understand biology, they have to understand chemistry clinical pharmacology, preclinical pharmacology. They need to understand the target space. Those are extremely conservative people as well, right? So they know what works, they know that most of the time it doesn’t work, and new shiny things usually do not work.
So when you present them with the I remember those presentations very clearly. Okay this is, ian Goodfellow paper on, handwritten characters. Here is Read paper on conditional generation of images, right? Here it’s like almost photorealistic. Look, this flower has the same number of petals.
And leaves as you as your desired condition, right? And it doesn’t happen in nature. Look, I just did the same with a molecule. So it’s like a big deal. Let’s just scale it up. Let’s collaborate. Let’s test it. Now oh, great. Beautiful picture is, yeah, it’s unbelievable. But no we don’t think it’s going to work in chemistry.
And until the time when one of the contract research organizations agreed to collaborate with us and test the molecules a little bit cheaper than it was originally intended and did a pilot with us, and they saw that, wow, you send the molecules that they don’t expect to work and they work.
For a target that is reasonably difficult to drug selectively, they’re like, okay, wow that’s, you’re onto something, right? So let’s let’s do a deeper dive, right? Then they a few CROs supported us otherwise we would have died. And very often even the investors, they come, learn, and they try to incubate something similar because they get more equity in the company.
And they usually learn from a few companies like you, even under CDA. That was very discouraging in the early days. And then they just flushed those companies with cash bringing the big names. Thanks. And then it’s easier to partner with pharma companies. So they do a few partnerships, but now they’ve failed to deliver anyway.
So it’s water under the bridge, but at that time it was difficult. Yeah.
Ray: Very interesting. Yeah. I’m sure the entire journey must have been had its ups and downs for sure. I guess I have a question. This is more on a high level. So with AI playing a more significant role now in healthcare and drug discovery, How do we ensure that these technologies remain ethical and unbiased?
And for the examples that you provided, chemistry and biology, the AI is basing its working knowledge, let’s say based on the data that we have existing, but there’s many things in science and healthcare that we still. have yet to understand in terms of biology, the brain and things like that.
How do we remain again, ethical and unbiased with all that?
Alex: So sure. So for that, you have very clear regulatory standards. So the beauty of biopharma is that everything is so regulated that regardless of how you discover the drug where you discovered it, who you are you have to be in the same playing space with everybody else.
And there are very specific rules, so the FDA is not going to give you any slack if your drug is not, has not been proven to be safe and also effective, right? And you actually don’t want to cut corners there. And they won’t let you originally, right? Hope they don’t. We didn’t get any slides.
I hope not either. Yeah, but At this point in time Once you submit to your application to the FDA to start a human clinical trial, It has to go through very rigorous review. And even if you use some, I don’t know, black magic to come up with a drug and it worked beautifully in many models, but it showed poor safety, they will not give you a good go ahead, right?
You would need to prove safety, and preferably to some extent efficacy before you start clinical trials. And then you have clinical trials, right? So clinical trials is what we live and die by as AI powered biotech. So your AI can be beautiful. It can perform miracles. You can, design drugs on the fly.
But later you will move with a speed of traffic, right? Because you have to demonstrate that the drug is safe. If you are addressing a chronic disease, you actually need to demonstrate that it’s safe and tolerable over an extended period of time and healthy volunteers. And then you test it in patients with certain diseases, and you need to do a double blind placebo controlled trial just like with any other technology.
From the ethical perspective, I think that I don’t see any problems when it comes to discovering development, right? Even if it is a black box very often many drugs that we take, we actually don’t know how they work. Go explain how metformin works, right? Some of the most popular drugs for for controlling your glucose, right?
Nowadays there are very clear targeted drugs GLP 1s, for example, that target a specific protein in your body and you at least to, Weight loss, but we still are struggling with understanding the complete biological picture. How much does it work in the brain? How much does it work in your peripheral system?
How does it affect the muscle? So we’re still learning, but things that we actually got used to, like even aspirin, right? So go ahead, like try to explain some of the oldest drugs. And usually when you submit your application to the FDA with a new targeted therapeutic, you would need to explain the mechanism in a much more rigorous way than most of the drugs that are approved to date, right?
And, have been in clinical practice for a long time. Even if you, and most of the time it will be explainable because it’s it has been developed for a specific goal. Usually we’re searching for a specific target, you’re designing a small molecule for a specific target. It’s actually even cleaner.
So you have a very clean path, right? Instead of saying, oh, I did a clinical trial and my drug works. I don’t know how, probably like this. But the most, but people will take it and the FDA would approve it because it works and it’s safe, right? Sometimes Yeah. especially for legacy drugs.
You don’t need to explain. Now you do.
Ray: Interesting. So it sounds like you feel like the current regulations, the FDA regulations are pretty robust and are ready for this increased AI, AI designed drug pipelines, I would say. Are there any laws or regulations you wish could change that may improve the innovation around AI designed or AI discovered drugs, for instance?
Alex: So actually, there are not that many AI drug discovery companies that managed to get like true AI discovery at AI design drugs into human clinical trials. Actually, outside of in silica, I don’t know any other like clean story, right? So novel target, novel molecule designed by AI. with papers published, right?
So some people decided to identify a target and then in license a molecule, right? So they skipped chemistry. Some people decided to go after a very well known, validated target that everybody knows, right? But it’s difficult to do chemistry, or you can do differentiated chemistry and make money.
And I decided to go after the non validated target. There are not that many, right? If you look around even, I’m pretty sure the FDA would struggle to find a really good list of those clean, AI generated drugs to either regulate or support, right? Support through through additional regulation.
Maybe sometimes regulation is actually positive, right? So they give you additional oversight, and that oversight may result in faster approvals, right? So I previously thought that before I started on drug discovery and before I started clinical trials, I was actually under the impression that the FDA was an inefficient mechanism, et cetera.
But once you get into the game and you understand that, they have certain amount of time to respond or a quotas. So they have been polished by the patient advocacy groups to the level where they cannot delay, right? And they have certain quotas for certain regulations certain rules internally where they have to respond.
And you understand, wow, my God, this is already a very well oiled machine, so you have to respect it. You have to respect the people who designed it, developed it, who work there. And we live and die by this agency, right? And usually it it treats everybody equally. And it treats those that have better drugs better, right?
So that’s they’re there for the patients. So of course it would have been great to have maybe additional support from the regulatory agencies. To discuss how we can accelerate that, but there are not that many companies that actually can accelerate. So my average time to preclinical candidate now is 13 months, right?
So it’s very quick. Average time to human clinical trial is about two years or even lower, right? From zero. So something that previously took let’s say four, four and a half, five years, six years, we can do it very quickly and with higher degree of novelty. And since we actually industrialized this process.
So my objective is to to license those drugs out to companies that are in need of novel therapeutics to develop. And they want to skip the discovery stage and build pipelines because they focus on clinical development. So you need to focus on the ultra high quality of the drug. So usually when pharma companies buy your drug, There are standards for the level of quality for your drug will be actually higher than they would have internally because you would have many more people looking at it, right?
So they have scientific teams to evaluate some level of due diligence will be massive if you want to sell a drug and I’ve sold several, right? We try to industrialize this process, and there are not that many companies that are able to do that. I don’t know of any other, actually. You have some traditional biotechs and some CROs that have the capability to move programs forward quickly and run them.
And it would be nice to actually work with a regulator to brainstorm, right? Where In which disease areas we could move faster cheaper, set records and maybe even explore specific geographic advantages of different regions. For example, If you are running a rare disease study, right?
It’s actually a better, it’s better to run it in China, for example, right? Or India or some other geography where there are, there is a lot of patients, right? So when there’s like 1. 5 billion people and it’s electronically very well connected. You can very quickly pick patients, right? And run clinical trials, observe it, right?
So I think mobile collaboration could help a lot. And we could try to set some records, right? Let’s say, can we get a drug approved in five years? Yes or no? For a rare disease that has no cure, people are dying. Let’s try to ensure that they don’t, right? We could try and set some records.
Absolutely. I think within the current framework, we need to build up more evidence. That this capability is there just like SpaceX, right? So I like to compare and silico to SpaceX, because when you are asking a company, okay, what’s your competitive advantage? What is the secret sauce? Is it the engine?
Is it your chemistry? Is it your biology? Is it your? People, is it your factory, or is it your automated lab, and you say it’s the ability to launch drugs into human clinical trials from zero. So it’s, you have to be good at everything. It has to be a well welded machine, constantly improve all the different steps and pieces optimize.
But before that, you just need to show that you can get to orbit, right? Then you can deliver the payload and then you just need to show that you are consistent. And then, start doing missions, right? So that’s what we are trying to do it in Silicon.
Ray: That’s amazing. I appreciate that response and you mentioned how there are certain regions that might be better fit for clinical trials, like China India.
In addition to those, are there other regions that you might think are ready, more ready to adopt AI for, biotech innovation and things like this?
Alex: I always like to think about what I can do for Africa, for example, right? And Southeast Asia like outside the very developed countries where people might not have the same kind of birth privileges we got I was not born into wealth, right?
But a lot of people think that They are poor in developed countries, but you have to actually travel to see what poor looks like, right? And I think AI may help those regions in a very dramatic way, right? Because now on your cell phone, you’ve got the capabilities of a university professor working for you educating you, right?
So it’s all about democratization of this technology. And I think that many of those countries that were previously considered to be. Or an uneducated can benefit from this explosion of intelligence, explosion off educational capabilities. All we need to ensure is that they all have cell phones, and instead of getting addicted to, viral videos and, video games, etc. So they actually start wasting time. They, instead, they realize that there is a benefit in learning and doing something extremely practical and high utility, high impact. So it’s also a little bit of motivation and education direction. So my dream was to go into those developing nations and build drug discovery pro programs.
And also for countries that are extremely wealthy for example, in the Middle East they have never discovered a drug. So drug discovery is a molecular casino where you actually have to bet on many different targets, right? Many different on many different spaces.
And that’s a lot, right? And every time the roulette moves and you win, it’s it’s not the immediate win. You have to roll again, right? So double down, roll again. And only in phase three, you realize, okay now the payout is great, right? So then you can Pay for your entire betting activity.
But this takes decades, right? And many countries, even with a lot of cash, right? Countries that are rich in oil, and that are pumping oil from the ground they’re looking to diversify and looking to get off the kind of oil needle. But somehow they don’t bet on biotech, right? Because their wildest dream is to run, a phase two human clinical trial as an extension of a big pharma company.
They usually don’t really think about how do we discover a drug locally. And when they get to that level, their first question is, Oh, how do we build the infrastructure to do that, right? We need to have chemistry labs biology labs, etc. Actually you don’t need that, right? You just need to have a few really smart guys who know, or gals who know how to discover drugs, how the industry works, right?
And apply AI to and some money. To discover a drug, synthesize tests anywhere in the world, there are contract research organizations for that. So build the expertise and showcase that you can discover the drug in your country. So and run the program. Some of the companies, look, Summit Therapeutics so 108 people, 108 employees.
No dramatic infrastructure. $21 billion in market value right now on the market, right? Because one of their bispecifics demonstrated significant efficacy by a partner Akio in China in a clinical trial, right? Boom, $21 billion, Viking Therapeutics, right? So some are 28 people, right?
According to the financials. And 7. 5 to 10 billion dollars in market value on public, publicly traded, right? So it’s not the game where you need to have a lot of infrastructure and people. You just need to have a few very smart people and placing bets, right? So if you don’t place those bets, you won’t be able to play the game.
And my job is also to go and educate those countries, right? Most of them may think that they need to spend decades to put the infrastructure in place. But you cannot compete with the infrastructure that developed countries already placed in place, right? They spent 1. 2, 1. 5 trillion dollars on that.
You’ve got tens of thousands of people and robots working there providing services. Our AI now can convert money into drugs. That’s amazing. rich countries and hopefully provide some tools for developing countries to start playing this game.
Ray: It is quite incredible how fast everything is moving, but I only have a couple minutes left with you.
So I’m going to ask you two final questions quick questions and then we’ll adjourn this meeting. So what are you most excited about when you look 10 to 20 years into the future of biotech and AI? So this is quite into the future.
Alex: 10 to 20 years, that’s a long time from now, so I’m extremely excited about of course, the generative approaches plus robotics that we are working on.
Nowadays, you can do automated, goal oriented experimental planning, and multi agent systems and robots will perform. experiments based on your ultimate objectives. I’m very excited about quantum computing right now. So I think that the next ten years, we’re currently probably in the age as generative was 2016.
So there are some early birds, but people don’t believe that it’s gonna work. But it will work, right? So sorry guys, we even synthesized and tested the first molecule generated on a quantum computer. Done, right? So POC is there, so it will scale. You have to believe in that, right? And as quantum computing scales, we will be able to create comprehensive life models, simulations over the entire world.
I hope that we are not a simulation to find some drug, right? That would be like really bad, especially if you are not even like non human character. And we’re not aware of it, right? Yeah you don’t want to be aware of that, right? Remember this freaking morning? Teaches you a lot, but I think that Those are the three directions that I am extremely excited about and I think that regenerative medicine, right?
So the ability to grow new organs organ systems maybe possibly in the future in 20 years. Maybe entire bodies are brainless, right? So there are multiple groups looking into that to provide, supply of young healthy organs that are biocompatible in the most ethical way. So I think 20 years, that’s going to be the direction, right?
Because the best the best way to cure, let’s say, kidney cancer. New kidney, why not?
Ray: That’s very exciting. And I hope you’re right. This is the final question. We’ll make it quick. What is the one thing everyone can do today to live a little bit longer, a little bit better?
Alex: You have to strategically convince yourself that you can live, let’s say 120.
Ray: So 120 is like the target.
Alex: yeah. So psychological aging is the most plastic. We’re published on that. If you convince yourself that you can live significantly longer than your parents and you will. Unless something dramatic happens you will act as a younger individual you will actually be healthier.
Ray: I appreciate that Alex.
Thank you so much for your time today. I think the audience has learned a lot from this conversation. If you did enjoy this, please share and subscribe to help us reach more people. Thank you very much. Great to be with you.
Thank you for reading!
Thank you for watching, listening, and/or reading this interview with Alex and Ray. If you enjoy this content, please like, share, and subscribe on your favorite social platform so we can reach more like you. Check out Vibe Bio resources for additional content.
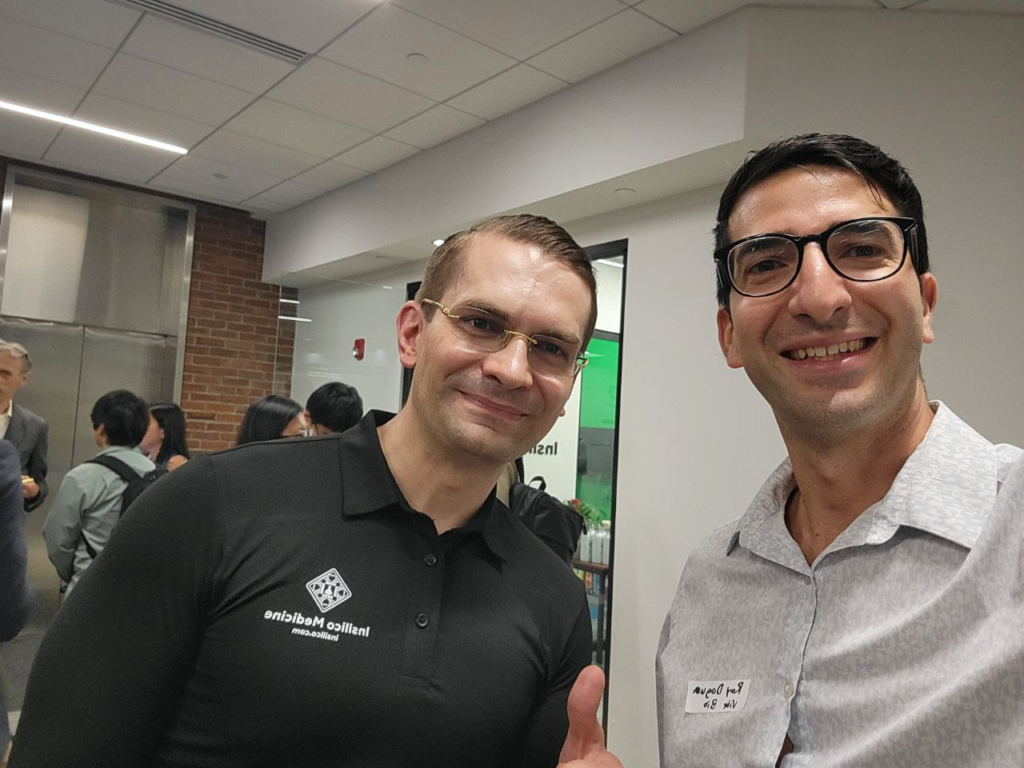
Ray and Alex at the new Insilico Medicine office in Cambridge, MA.